Overview
Machine learning (ML) systems are achieving remarkable performances at the cost of increased complexity. Hence, they become less interpretable, which may cause distrust. As these systems are pervasively being introduced to critical domains, such as medical image computing and computer assisted intervention (MICCAI), it becomes imperative to develop methodologies to explain their predictions. Such methodologies would help physicians to decide whether they should follow/trust a prediction or not. Additionally, it could facilitate the deployment of such systems, from a legal perspective. Ultimately, interpretability is closely related with AI safety in healthcare.
However, there is very limited work regarding interpretability of ML systems among the MICCAI research. Besides increasing trust and acceptance by physicians, interpretability of ML systems can be helpful during method development. For instance, by inspecting if the model is learning aspects coherent with domain knowledge, or by studying failures. Also, it may help revealing biases in the training data, or identifying the most relevant data (e.g., specific MRI sequences in multi-sequence acquisitions). This is critical since the rise of chronic conditions has led to a continuous growth in usage of medical imaging, while at the same time reimbursements have been declining. Hence, improved productivity through the development of more efficient acquisition protocols is urgently needed.
This workshop aims at introducing the challenges & opportunities related to the topic of interpretability of ML systems in the context of MICCAI.
Scope
Interpretability can be defined as an explanation of the machine learning system. It can be broadly defined as global, or local. The former explains the model and how it learned, while the latter is concerned with explaining individual predictions. Visualization is often useful for assisting the process of model interpretation. The model’s uncertainty may be seen as a proxy for interpreting it, by identifying difficult instances. Still, although we can find some approaches for tackling machine learning interpretability, there is a lack of formal and clear definition and taxonomy, as well as general approaches. Additionally, interpretability results often rely on comparing explanations with domain knowledge. Hence, there is need for defining objective, quantitative, and systematic evaluation methodologies.
Covered topics include but are not limited to:
- Definition of interpretability in context of medical image analysis.
- Visualization techniques useful for model interpretation in medical image analysis.
- Local explanations for model interpretability in medical image analysis.
- Methods to improve transparency of machine learning models commonly used in medical image analysis.
- Textual explanations of model decisions in medical image analysis.
- Uncertainty quantification in context of model interpretability.
- Quantification and measurement of interpretability.
- Legal and regulatory aspects of model interpretability in medicine.
Program
The program of the workshop includes keynote presentations of experts working in the field of interpretability of machine learning. A selection of submitted manuscripts will be chosen for short oral presentations (10 minutes + 3 minutes Q&A) alongside the keynotes. Finally, we will have a group discussion which leaves room for a brainstorming on the most pressing issues in interpretability of machine intelligence in the context of MICCAI.
Final program:
- 15:00 – 16:00: Keynote: "Overview of Interpretability methods and Interpretability Beyond Feature Attribution, TCAV" by Been Kim.
- 16:00 – 16:40: Accepted contributions:
- "Regression Concept Vectors for Bidirectional Explanations in Histopathology" by Graziani et al. (HES-SO Valais) - (Awarded as best paper) (preprint)
- "Visualizing Convolutional Neural Networks to Improve Decision Support for Skin Lesion Classification" by Van Molle et al. (U Ghent) (preprint)
- "Automatic brain tumor grading from MRI data using convolutional neural networks and quality assessment" by Pereira et al. (U Minho) (preprint)
- 16:40 – 17:00: Refreshments.
- 17:00 – 17:45: Keynote: "Recent Advances in Our Ability to Explain the Predictions of Complex Models" by Scott Lundberg.
- 17:45 – 18:25: Accepted contributions:
- "Collaborative Human-AI (CHAI): Evidence-Based Interpretable Melanoma Classification in Dermoscopic Images" by Codella et al. (IBM Research) - video (preprint)
- Towards complementary explanations using Deep Neural Network" by Silva et al. (INESC TEC Porto) (preprint)
- "Explainable Artificial Intelligence: How Users Perceive Content-based Image Retrieval for Skin Lesion Images" Sadeghi et al. (Simon Fraser University) (preprint)
- 18:25 – 19:00: Group discussion and wrap-up.
Keynote speakers
-
Been Kim, Google Brain, USA.
Title: Overview of Interpretability methods and Interpretability Beyond Feature Attribution, TCAV
-
Scott Lundberg, University of Washington, USA.
Title: Recent Advances in Our Ability to Explain the Predictions of Complex Models
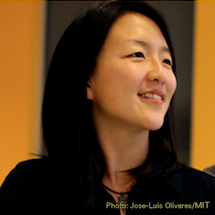
Abstract: In the first part of the talk, I will provide an overview of interpretability methods in deep learning and their limitations. In the second part, I will talk about a couple my recent work including Testing with concept activation vectors (TCAV). The interpretation of deep learning models is a challenge due to their size, complexity, and often opaque internal state. In addition, many systems, such as image classifiers, operate on low-level features rather than high-level concepts. To address these challenges, we introduce Concept Activation Vectors (CAVs), which provide an interpretation of a neural net's internal state in terms of human-friendly concepts. The key idea is to view the high-dimensional internal state of a neural net as an aid, not an obstacle. We show how to use CAVs as part of a technique, Testing with CAVs (TCAV), that uses directional derivatives to quantify the degree to which a user-defined concept is important to a classification result--for example, how sensitive a prediction of “zebra” is to the presence of stripes. Using the domain of image classification as a testing ground, we describe how CAVs may be used to explore hypotheses and generate insights for a standard image classification network as well as a medical application.
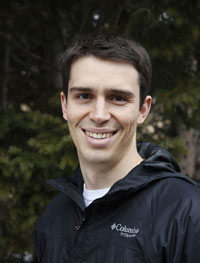
Abstract: Understanding why a machine learning model makes a certain prediction can be as crucial as the prediction’s accuracy. However, the highest accuracy for large modern datasets is often achieved by complex models that even experts struggle to interpret, such as ensemble or deep learning models, creating a tension between accuracy and interpretability. In response, various methods have recently been proposed to help interpret the predictions of complex models, but it is often unclear how these methods are related and when one method is preferable over another. I will review a variety of recent approaches and discuss our work, motived by operating room decision support, that connects many of these approaches to each other, and to formal guarantees.
Paper submission
Authors should prepare a manuscript of 8 pages, including references. The manuscript should be formatted according to the Lecture Notes in Computer Science (LNCS) style. All submissions will be reviewed by 3 reviewers. The reviewing process will be single-blinded. Authors will be asked to disclose possible conflict of interests, such as cooperation in the previous two years. Moreover, care will be taken to avoid reviewers from the same institution as the authors. The selection of the papers will be based on their relevance for medical image analysis, significance of results, technical and experimental merit, and clear presentation.
We intend to join the MICCAI Satellite Events joint proceedings, and publish the accepted papers as LNCS. We are also considering making the pre-print of the accepted papers publicly available.
Click here to submit your paper.
Important dates
- Opening of submission system (Mid May).
- Submission deadline (18th June).
- Reviews due (9th July).
- Notification of acceptance (13th July).
- Camera-ready papers (20th July).
Organizers
- Mauricio Reyes, University of Bern, Switzerland.
- Carlos A. Silva, University of Minho, Portugal.
- Sérgio Pereira, University of Minho, Portugal.
- Raphael Meier, University of Bern, Switzerland.
Technical Committee
- Ben Glocker, Imperial College, United Kingdom.
- Bjoern Menze, Technical University of Munich, Germany.
- Carlos A. Silva, University of Minho, Portugal.
- Christoph Molnar, Ludwig Maximilian University of Munich, Germany.
- Dwarikanath Mahapatra, IBM Research, Australia.
- Mauricio Reyes, University of Bern, Switzerland.
- Raphael Meier, University of Bern, Switzerland.
- Richard McKinley, University of Bern, Switzerland.
- Sérgio Pereira, University of Minho, Portugal.
Photos of iMIMIC 2018
Photos of the event.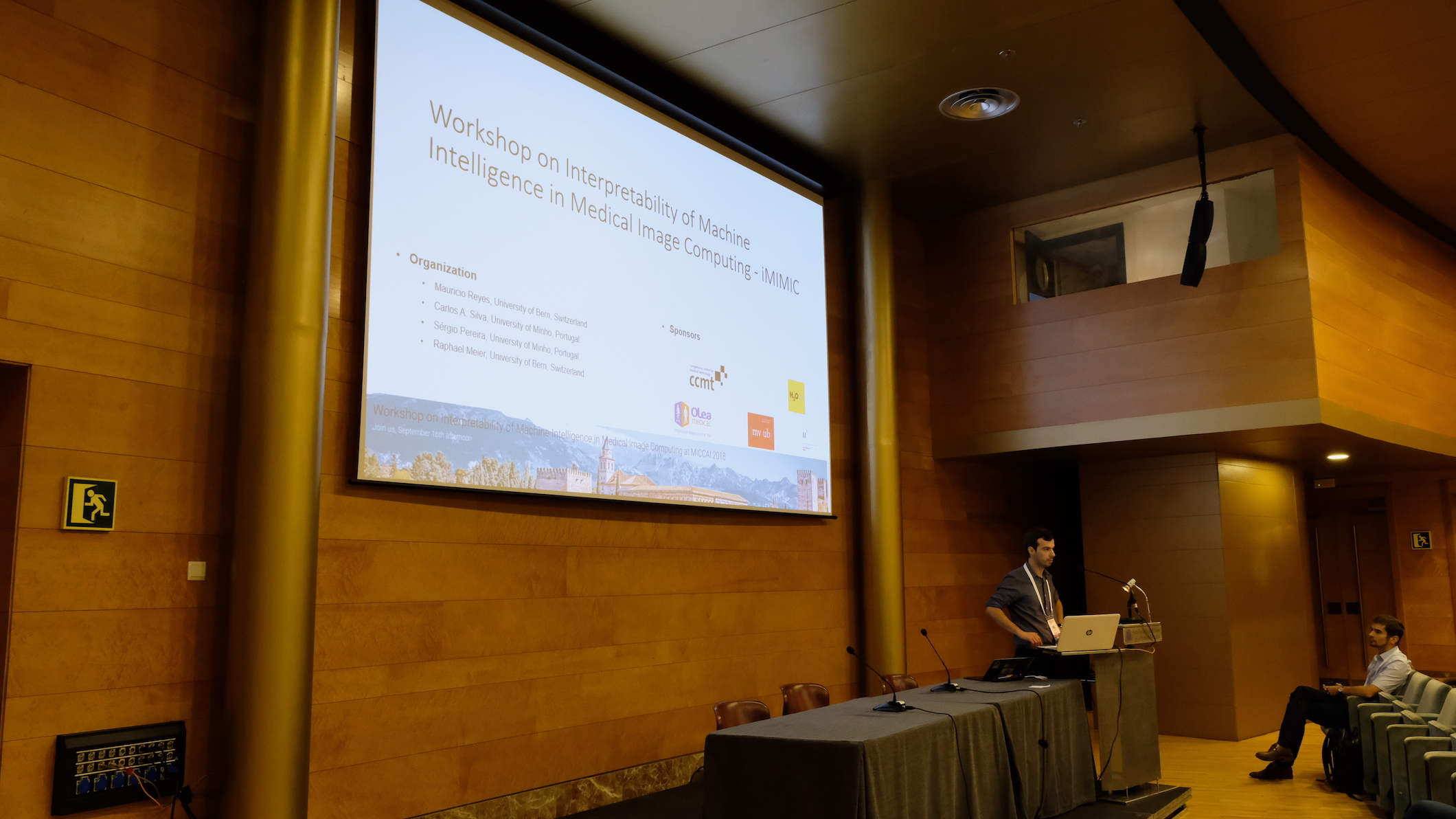
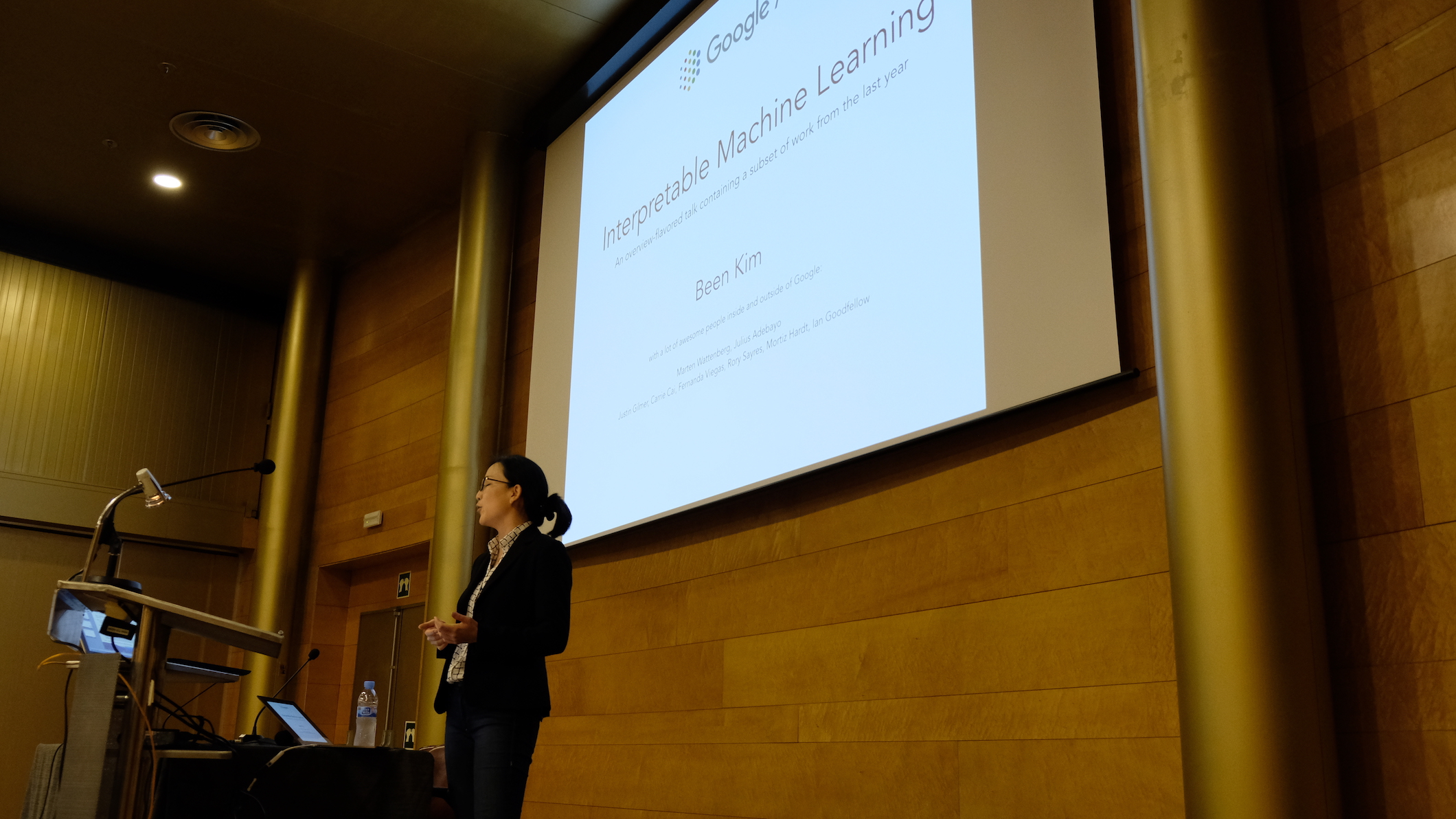
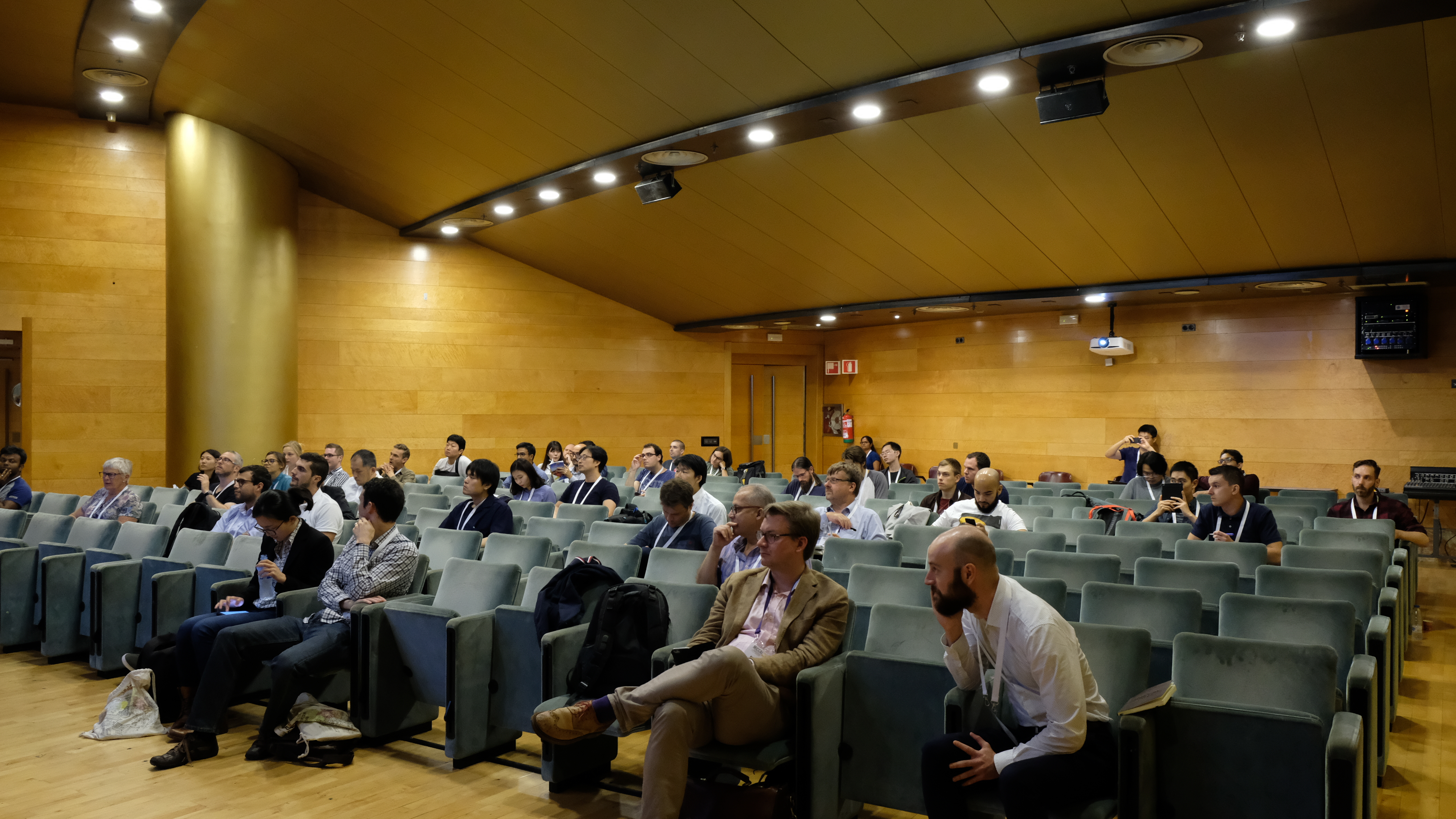
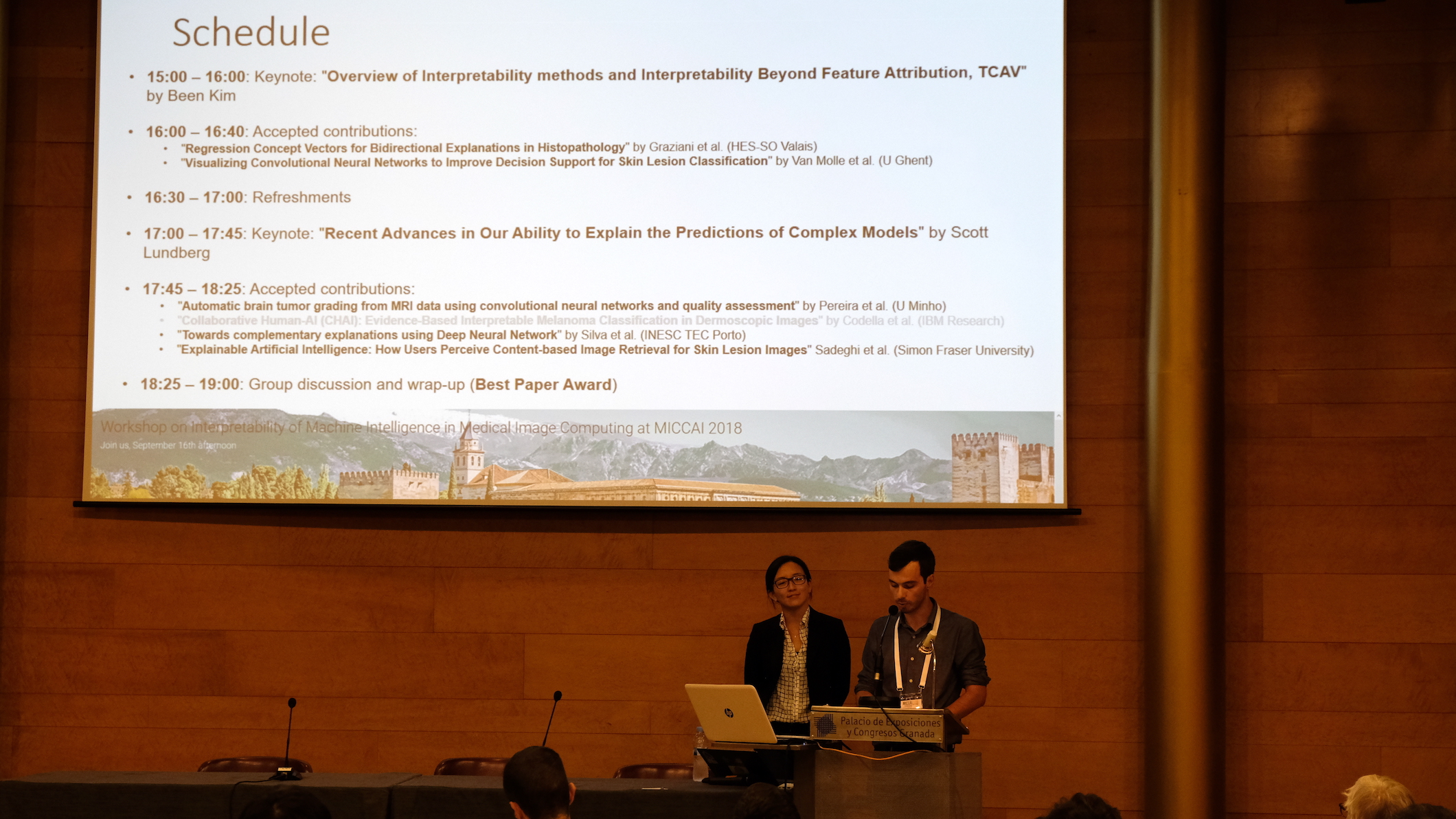
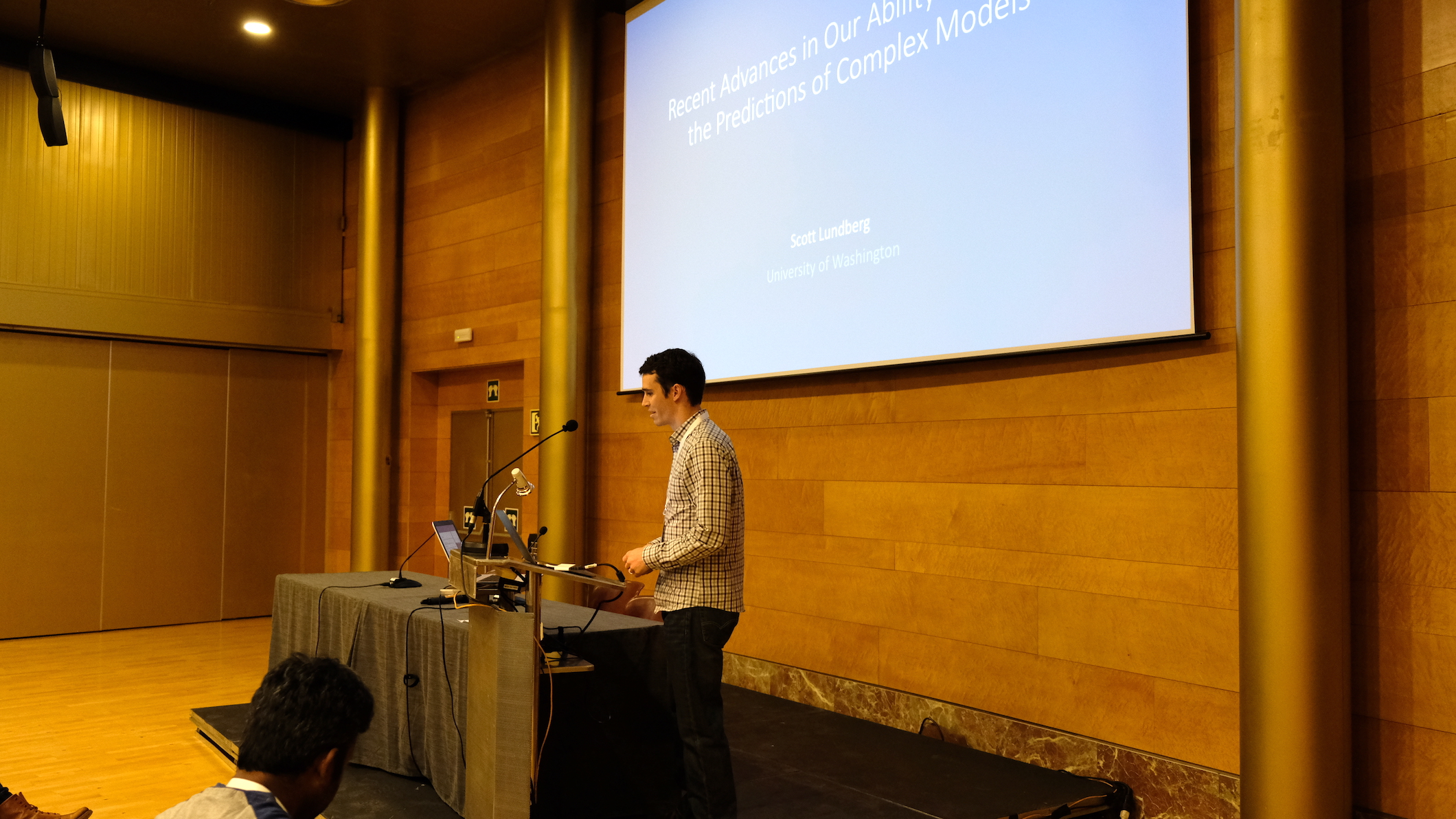
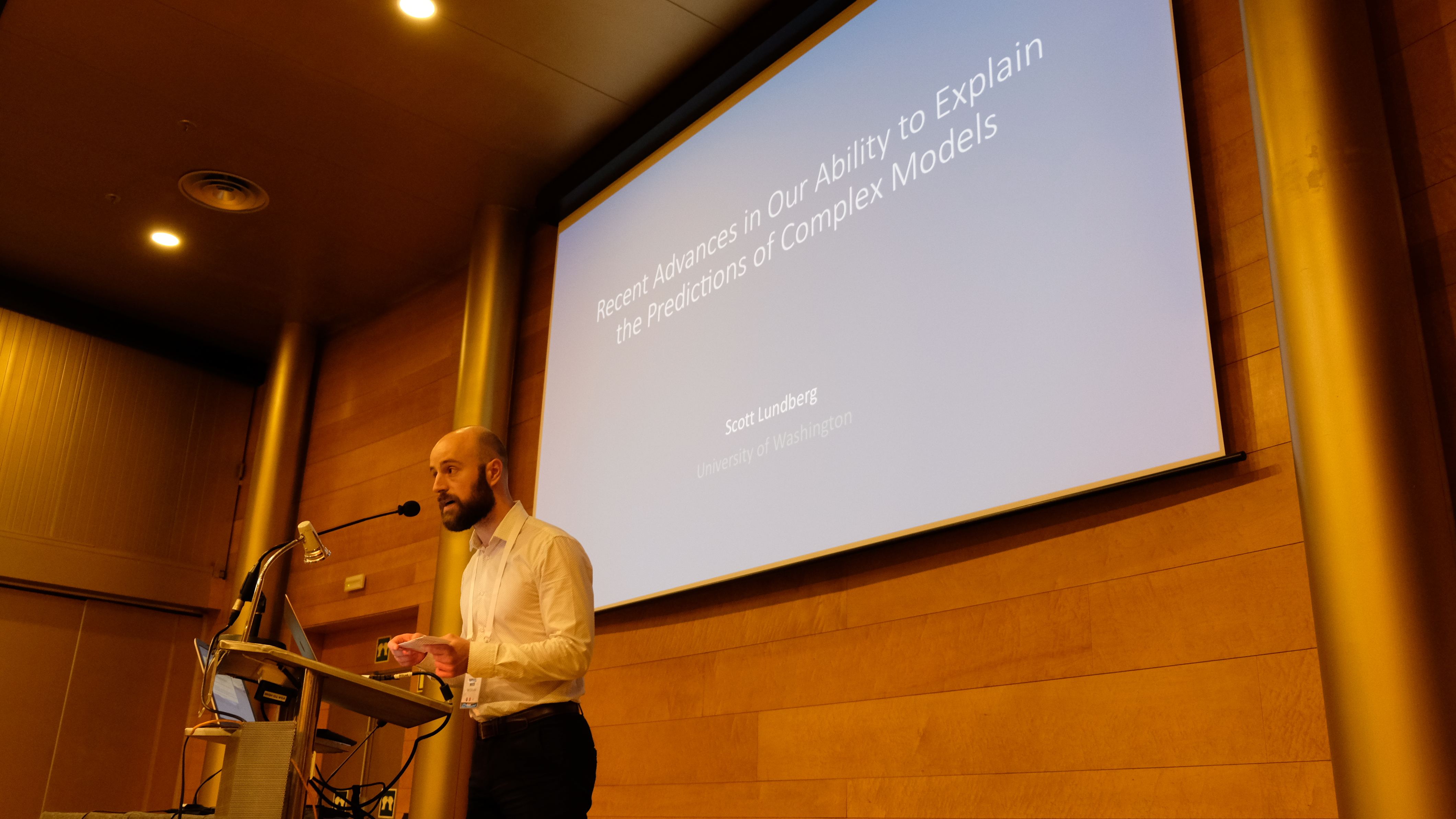